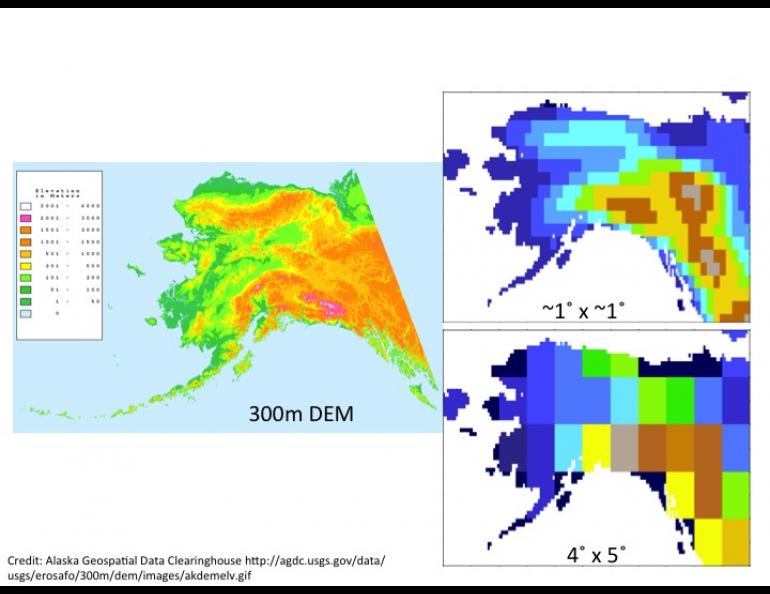
Where do climate projections come from?
In Alaska, our lives revolve around the weather. When it comes to predicting conditions like temperature, snow and rain, the best glimpse into the future comes from climate models.
But standard climate models are very broad—looking at how global climate will be affected by things like escalating carbon dioxide emissions.
In a land of permafrost, icefields, massive mountain ranges and rainforest, a more nuanced prediction is helpful. For example, will it get rainier in the North Slope over the next few decades? When might Southcentral’s epic snow dumps turn to rain?
Steph McAfee recently produced a dataset that imposes high-resolution data from weather stations onto global climate models of the future to try to predict Alaska’s climate with more precision. It gives detailed projections for snow in different regions of the state over the next century.
“It brings the data to a spatial scale that is more accessible and useful,” says McAfee, a climatologist with the Scenarios Network for Alaska and Arctic Planning (SNAP), a climate change planning group at the University of Alaska Fairbanks.
The technique is called downscaling. Picture Alaska divided into a grid of big cells of data on future global climate (approximately 225 kilometers by 95 kilometers per cell). Each big cell represents a single value—for example, a predicted temperature rise of 1 degree Fahrenheit over 30 years. Then you overlay a much tighter grid (2 kilometer-by-2-kilometer cells) of real-world data on temperature and precipitation. Now apply the value from the big cell onto each unique smaller cell within it (i.e. add 1 degree). This is what today’s climate could look like in 30 years.
The big cells come from global climate models produced by climatology groups. SNAP selected five well-known models based on their accuracy in predicting historical climate and their applicability to the arctic.
The small cells come from pre-packaged analyses of weather station data, including average monthly temperature and geographic information such as elevation, aspect and proximity to a coast or large body of water–all factors that influence snow formation.
A big cell covering the Kenai Peninsula, for instance, gives an average temperature for the whole box. Yet Kenai contains mountains and icefields, which would be colder than the rest of the peninsula.
“Having those elevational gradients expressed is important. A coarse resolution model might not know there are mountains there. It might not know you have snowfall there in June.”
Broadscale climate models have been fairly accurate in predicting global climate change over the past few decades. Yet they are incredibly complex, relying on many hard-to-predict factors such as carbon emissions, solar energy levels, volcanoes and aerosols produced from power plants and other sources.
Downscaling these models with real-world data adds a new layer of uncertainty.
For example, the high-resolution climate data comes in monthly averages and doesn’t include information on rain or snow. To get that, McAfee consulted weather stations across the state to see how many days it rained versus snowed, and calculated a relationship between precipitation and temperature. Then she incorporated that relationship into the higher-resolution grid to predict snowfall across the state.
Still, you can never be sure what fell from the sky.
“Last October in Anchorage, we got most of our precipitation during the first week. It was relatively warm and it rained. Then it got really cold and clear and we didn’t get much if any snow,” McAfee says. “By looking at the monthly averages, you would have no idea that almost all the precipitation was rain.”
Also, because there are only so many weather stations (this study used 100), that data has to be expanded to cover all the 2-by-2-kilometer cells in the state, which weakens its accuracy.
While projected snow varies greatly across the five climate models, one thing is clear: milder climates will lose the most snow, and fastest.
In an aggressive warming scenario, Southwest and Bristol Bay would see almost exclusively rain by the end of the century. While the North Slope and Interior may get more rain in the shoulder seasons, snow will still dominate in the winter.
The data can be used by anyone interested in future snow levels--public land planners, wildlife managers or the ski industry.
“It may not be perfect but it will help some people with some of their questions and identify where we might need more advanced techniques or different methods,” McAfee said.
The snow data set includes nine decades worth of monthly outlooks. It can be found at http://www.snap.uaf.edu/data.php